Introduction:
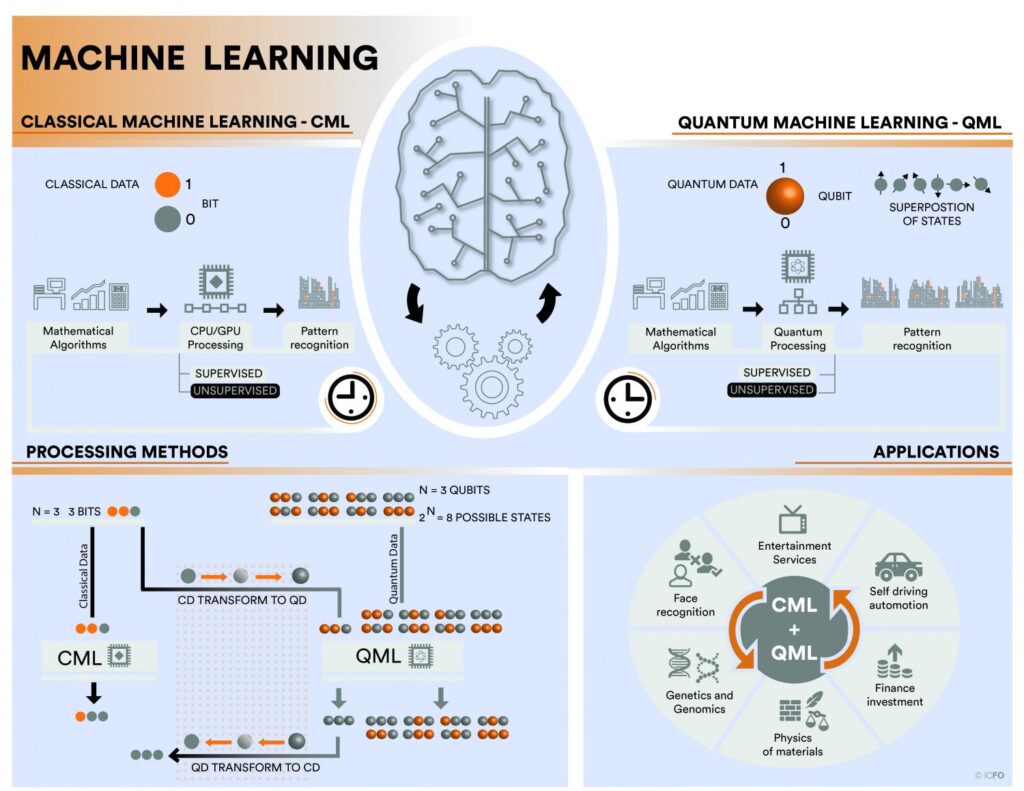
In the ever-evolving landscape of technology, the convergence of quantum computing and machine learning has sparked a revolution. Quantum machine learning (QML) stands at the forefront of this revolution, promising to revolutionize various industries by harnessing the power of quantum mechanics to enhance traditional machine learning algorithms. In this article, we’ll delve into the intricacies of quantum machine learning, exploring its principles, applications, challenges, and potential impact on the future of data science.
Understanding Quantum Machine Learning:
Quantum machine learning represents the intersection of two cutting-edge fields: quantum computing and machine learning. While classical computers process data using bits—binary units of 0s and 1s—quantum computers leverage quantum bits or qubits, which can exist in multiple states simultaneously due to the principles of superposition and entanglement. This unique property enables quantum computers to perform complex calculations exponentially faster than classical computers, particularly for certain types of problems.
In quantum machine learning, researchers aim to exploit quantum phenomena to enhance various aspects of traditional machine learning algorithms. These enhancements could lead to significant advancements in data processing, pattern recognition, optimization, and predictive modeling tasks.
Applications of Quantum Machine Learning:
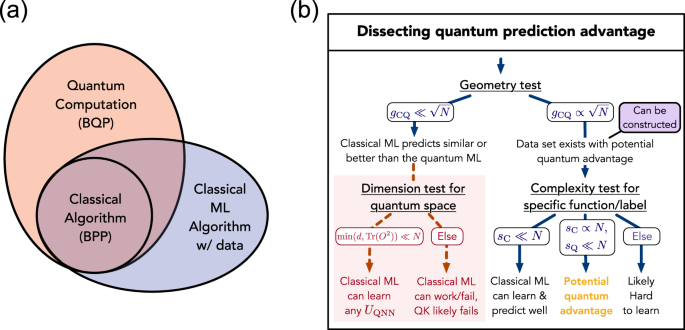
The potential applications of quantum machine learning span across numerous industries, including but not limited to:
- Drug Discovery: Quantum machine learning can accelerate the process of drug discovery by simulating molecular interactions and predicting the efficacy of potential drug compounds with unprecedented speed and accuracy.
- Financial Modeling: Quantum algorithms can optimize investment strategies, risk assessment models, and portfolio management techniques, providing more reliable predictions in volatile financial markets.
- Supply Chain Optimization: Quantum machine learning algorithms can optimize supply chain logistics, minimizing costs, reducing inefficiencies, and enhancing overall operational efficiency.
- Image and Speech Recognition: Quantum algorithms have the potential to enhance image and speech recognition systems, enabling more accurate and efficient processing of multimedia data.
- Cryptography and Cybersecurity: Quantum machine learning techniques can strengthen cryptographic protocols and cybersecurity measures, safeguarding sensitive data against quantum threats posed by quantum computers.
Challenges and Limitations:
Despite its tremendous potential, quantum machine learning faces several challenges and limitations.
- Quantum Hardware Constraints: Building reliable and scalable quantum hardware remains a significant challenge. Quantum computers are highly sensitive to environmental disturbances, and maintaining the coherence of qubits for extended periods is technically demanding.
- Algorithm Design: Designing quantum algorithms that outperform their classical counterparts for real-world applications requires innovative approaches and a deep understanding of both quantum computing and machine learning principles.
- Data Encoding and Decoherence: Converting classical data into a quantum format and mitigating decoherence—the loss of quantum information due to interactions with the environment—are ongoing research areas in quantum machine learning.
- Access to Quantum Resources: Access to quantum hardware and expertise in quantum computing is limited, hindering the widespread adoption and experimentation of quantum machine learning techniques.
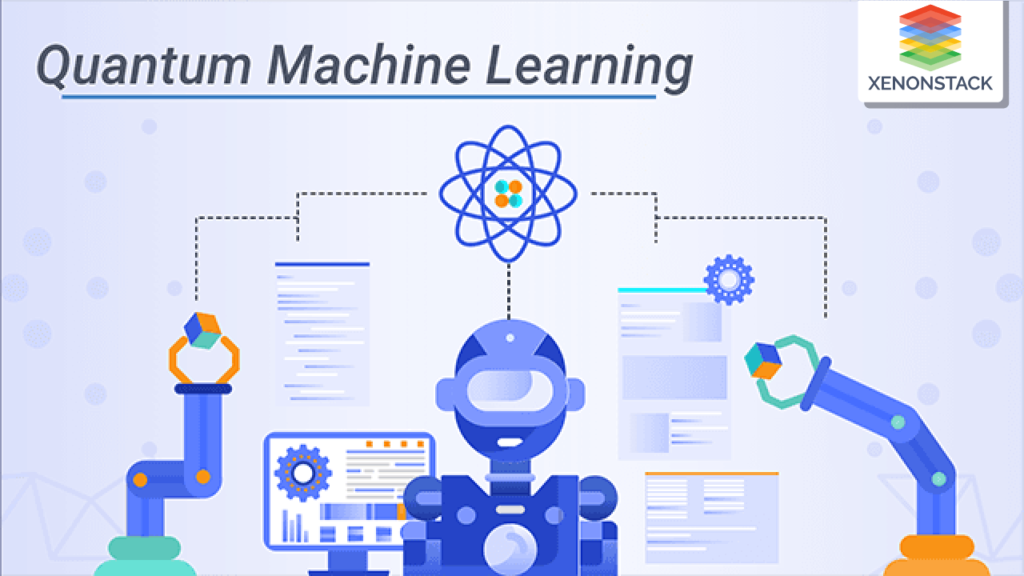
Future Prospects:
Despite the challenges, the future of quantum machine learning appears promising. As quantum hardware continues to advance, researchers are making significant strides in developing novel quantum algorithms and applications. Collaborations between experts in quantum computing, machine learning, and various industry domains are driving innovation and expanding the frontier of quantum machine learning.
Conclusion:
Quantum machine learning represents a groundbreaking approach to solving complex computational problems by leveraging the principles of quantum mechanics. While still in its infancy, this interdisciplinary field holds immense promise for revolutionizing data science and transforming industries across the globe. As researchers continue to unravel the mysteries of quantum computing and refine quantum machine learning techniques, we can anticipate a future where quantum-powered algorithms redefine the boundaries of what’s possible in data analysis, prediction, and decision-making.