Introduction:
In the realm of cutting-edge technology, two fields stand out for their promise of revolutionizing the way we understand and interact with the world: Quantum Computing and Artificial Intelligence (AI). While each has made significant strides independently, their convergence presents a paradigm shift with profound implications across industries. This article delves into the symbiotic relationship between quantum computing and AI, exploring the potential synergies and the transformative impact they hold for the future.
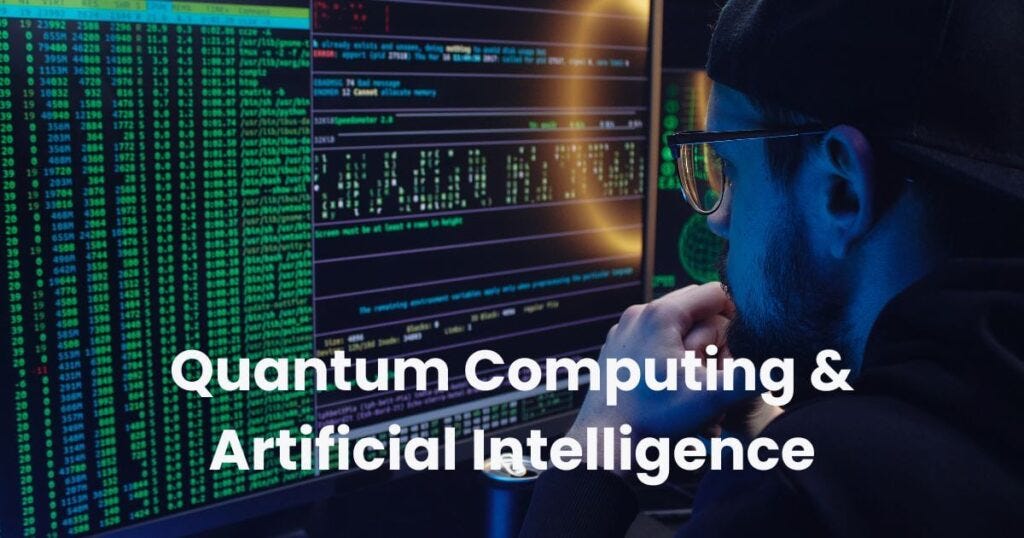
Understanding Quantum Computing:
Quantum computing harnesses the principles of quantum mechanics to process information in ways fundamentally different from classical computing. Classical computers rely on bits, which can represent either a 0 or a 1, while quantum computers leverage quantum bits or qubits, which can exist in multiple states simultaneously due to superposition and entanglement.
This inherent parallelism enables quantum computers to perform complex calculations exponentially faster than classical computers, making them particularly well-suited for tackling optimization problems, cryptography, and simulating quantum systems.
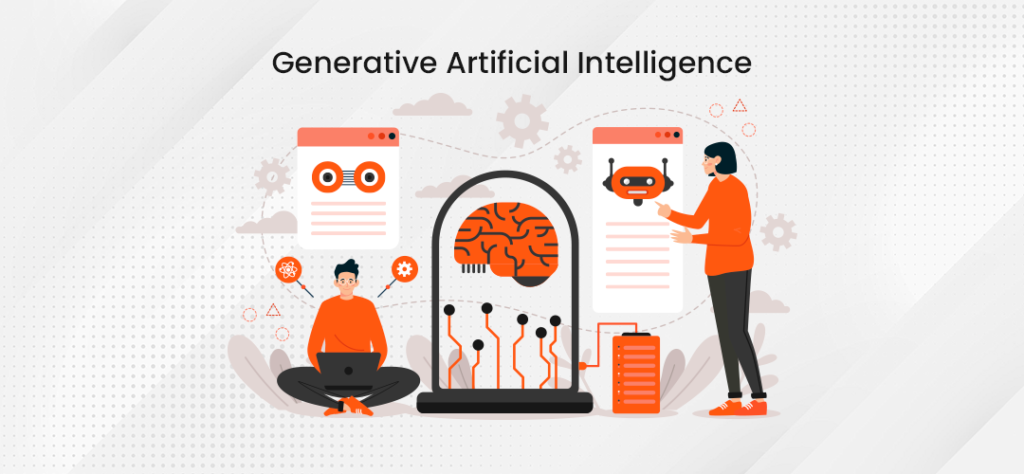
Artificial Intelligence and Machine Learning:
Artificial Intelligence encompasses a range of technologies aimed at enabling machines to perform tasks that typically require human intelligence. Machine Learning (ML), a subset of AI, focuses on developing algorithms that allow computers to learn from data and make predictions or decisions without explicit programming.
ML algorithms power a myriad of applications, from recommendation systems and natural language processing to image recognition and autonomous vehicles. As data volumes continue to grow exponentially, the demand for more powerful computing resources to train and deploy increasingly sophisticated AI models escalates.
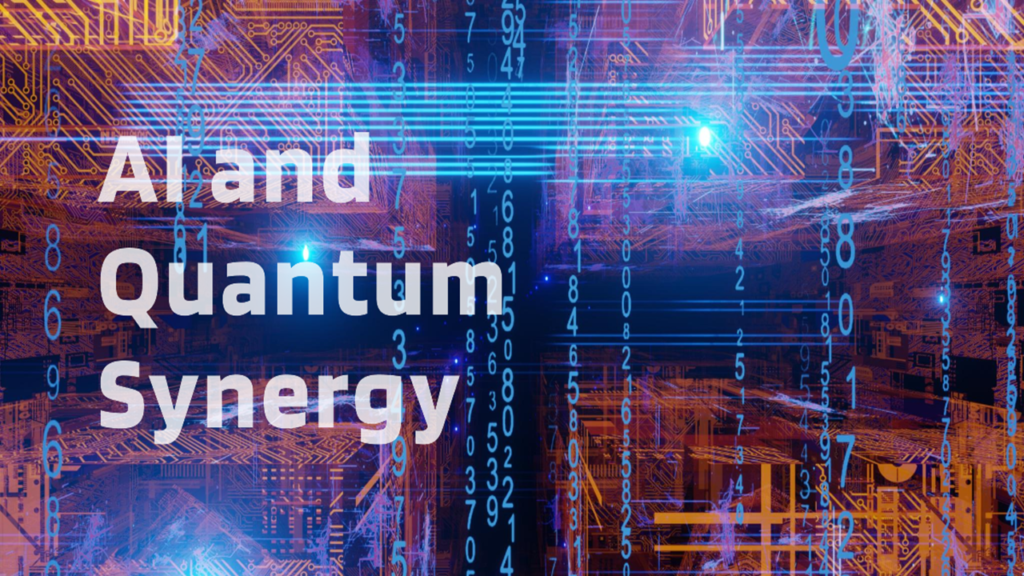
Synergies Between Quantum Computing and AI:
The marriage of quantum computing and AI holds immense potential to address some of the most complex and computationally intensive challenges facing AI today. Several key areas highlight the synergistic relationship between these fields:
- Accelerated Training of AI Models:
Training deep learning models on large datasets is a resource-intensive process that can take days or even weeks using conventional hardware. Quantum computing’s ability to process vast amounts of data and perform complex optimization tasks in parallel could dramatically reduce training times, speeding up AI innovation. - Enhanced Pattern Recognition:
Quantum computing’s inherent capacity for parallelism and complex probabilistic calculations could improve AI algorithms’ ability to recognize patterns and anomalies within datasets. This could lead to more accurate predictions and insights across various domains, including healthcare, finance, and cybersecurity. - Quantum Machine Learning Algorithms:
Researchers are exploring the development of quantum machine learning algorithms specifically designed to leverage the unique capabilities of quantum computers. These algorithms could outperform their classical counterparts in tasks such as clustering, classification, and dimensionality reduction, paving the way for new AI applications. - Quantum-Safe Cryptography:
As AI systems become increasingly integrated into critical infrastructure and communication networks, the need for robust cybersecurity measures grows. Quantum computing has the potential to render many traditional encryption methods obsolete due to its ability to quickly factor large numbers. However, quantum-safe cryptographic techniques, developed using quantum principles, could mitigate this threat and ensure the security of AI systems and data.
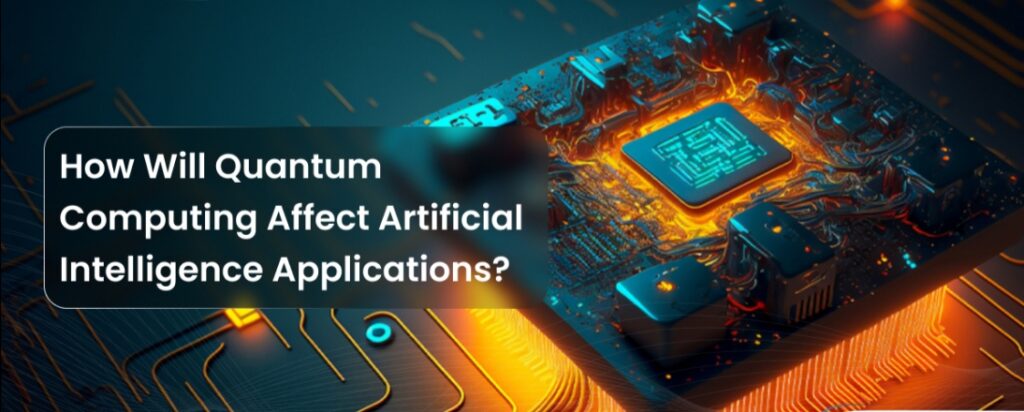
Challenges and Considerations:
Despite the immense promise, the convergence of quantum computing and AI also poses several challenges and considerations:
- Hardware Limitations:
Current quantum hardware is still in its infancy, with limited qubit coherence times and error rates. Overcoming these hardware constraints to build large-scale, error-corrected quantum computers capable of supporting complex AI workloads remains a significant hurdle. - Algorithmic Development:
Developing quantum machine learning algorithms requires a deep understanding of both quantum mechanics and machine learning principles. Researchers must bridge the gap between these disciplines to design algorithms that effectively harness the power of quantum computing while addressing the nuances of real-world datasets and applications. - Ethical and Societal Implications:
As AI capabilities continue to advance, ethical considerations surrounding privacy, bias, and the responsible use of AI become increasingly important. The integration of quantum computing may amplify these concerns, necessitating careful consideration of the potential societal impacts and regulatory frameworks.
Conclusion:
The convergence of quantum computing and artificial intelligence represents a groundbreaking frontier in technology, with the potential to revolutionize industries and transform society. By leveraging the inherent synergies between these fields, researchers and innovators can unlock new possibilities for tackling complex problems and driving innovation forward. As we continue to explore this exciting intersection, collaboration across disciplines and a commitment to ethical, responsible development will be crucial in shaping a future where quantum-powered AI technologies benefit humanity as a whole.