Introduction:
In the realm of cutting-edge technologies, Quantum Machine Learning (QML) emerges as a beacon of innovation, promising to revolutionize the landscape of artificial intelligence. This article delves into the transformative potential of Quantum Machine Learning, exploring recent advancements, the promise it holds for the future, and the challenges that lie ahead.
Understanding Quantum Machine Learning:
Quantum Machine Learning represents the fusion of quantum computing and machine learning, harnessing the unique properties of quantum systems to enhance learning algorithms’ efficiency and performance. At its core, QML aims to leverage quantum mechanics’ principles, such as superposition and entanglement, to process and analyze data in ways that classical computers cannot.
Advancements in Quantum Machine Learning:
1. Quantum Data Representation: QML introduces novel data encoding techniques that exploit quantum states to represent and manipulate information efficiently. Quantum feature maps, quantum circuits, and quantum embeddings offer powerful mechanisms for encoding complex datasets into quantum states, paving the way for enhanced learning and inference processes.
2. Quantum Variational Algorithms: Variational Quantum Algorithms (VQAs) form a cornerstone of QML, offering versatile frameworks for training quantum models using classical optimization techniques. VQAs leverage parameterized quantum circuits and classical optimization algorithms to adaptively tune quantum states, enabling efficient optimization of complex objective functions in machine learning tasks.
3. Quantum Neural Networks: Quantum Neural Networks (QNNs) represent a paradigm shift in neural network architectures, leveraging quantum principles to enhance learning and inference processes. QNNs exploit quantum entanglement and superposition to perform parallel computations, offering potential breakthroughs in areas such as pattern recognition, optimization, and generative modeling.
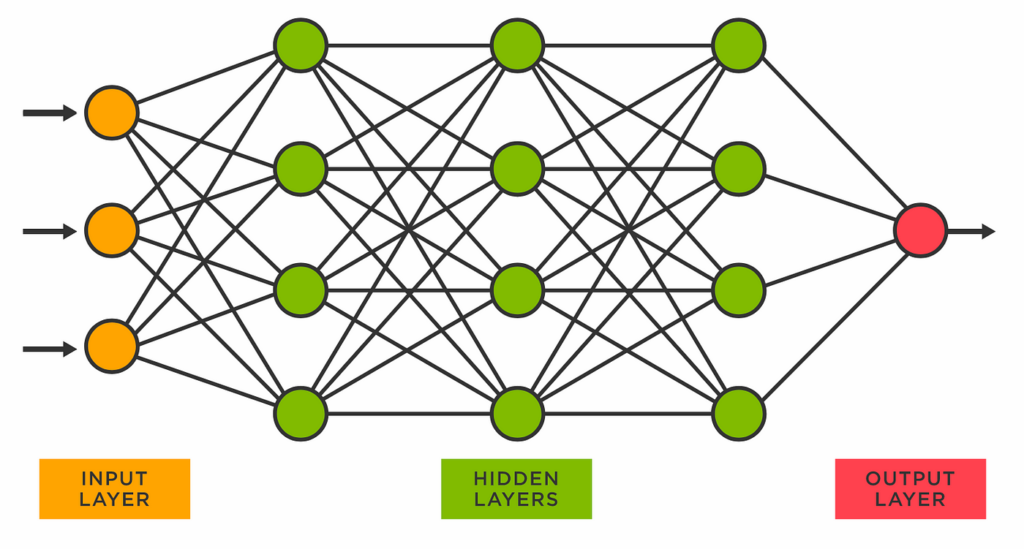
4. Hybrid Quantum-Classical Approaches: Many QML algorithms adopt hybrid quantum-classical approaches, combining the strengths of quantum processing with classical computation. Hybrid algorithms leverage quantum subroutines for specific tasks, such as quantum feature extraction or kernel functions, while employing classical algorithms for data preprocessing, optimization, and post-processing, achieving enhanced performance and scalability.
Challenges in Quantum Machine Learning:
1. Hardware Limitations: Current quantum hardware faces challenges such as qubit coherence times, gate fidelity, and error rates, limiting the scalability and reliability of quantum systems for machine learning applications. Overcoming these hardware constraints remains a significant hurdle in realizing the full potential of QML.
2. Algorithmic Development: Developing robust and scalable quantum machine learning algorithms requires interdisciplinary expertise in quantum physics, optimization, and machine learning. Bridging the gap between theoretical insights and practical implementations poses challenges in algorithm design, optimization, and validation.
3. Quantum-Classical Interface: Integrating quantum processing with classical computation necessitates efficient communication and synchronization between quantum and classical subsystems. Designing seamless interfaces and protocols for data exchange, parameter optimization, and error correction presents challenges in hybrid quantum-classical computing architectures.
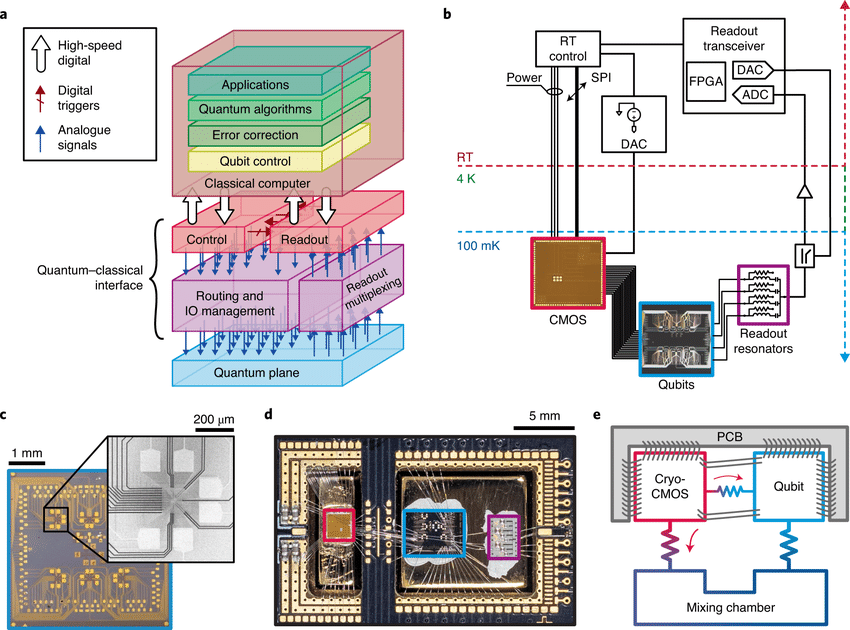
4. Ethical and Societal Implications: As QML technologies advance, ethical considerations surrounding privacy, bias, and transparency become increasingly pertinent. Responsible development practices, robust regulatory frameworks, and interdisciplinary collaboration are essential to address ethical and societal implications effectively.
Conclusion:
The promise of Quantum Machine Learning shines bright, offering unprecedented opportunities to push the boundaries of artificial intelligence and computational science. Despite the challenges that lie ahead, the relentless pursuit of innovation and collaboration across disciplines will drive QML’s advancement, unlocking new frontiers in machine learning, optimization, and data analysis. As we navigate this exciting journey, a commitment to ethical stewardship, responsible development, and inclusive dialogue will be paramount in harnessing QML’s transformative potential for the betterment of society.